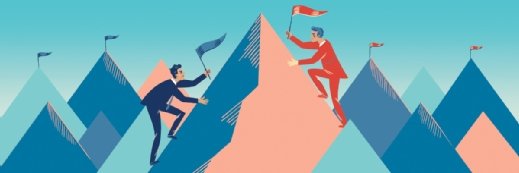
stock.adobe.com
MTA vs. MMM: What's the difference?
MTA and MMM help marketers improve their strategies, but MTA has a narrower focus. Key differences between these techniques include focus and data requirements.
As marketers struggle to link marketing activities to revenue, techniques like MTA and MMM can help bridge the gap.
Academics developed marketing mix modeling (MMM) in the 1960s to help organizations evaluate the effectiveness of their TV, print and radio marketing strategies. By the 2010s, customer journeys entered more digital channels, and tracking tools, like cookies, let marketers collect user data across the web. This more granular tracking data led to multitouch attribution (MTA), which helps organizations understand which touchpoints most significantly influence specific outcomes, such as sales and brand awareness.
MTA and MMM can both improve organizations' marketing strategies, but they differ in the following areas:
- Focus and perspective.
- Data requirements.
- Customer privacy.
- Timeframe.
Marketers should understand how these measurement techniques differ so they can implement the most effective campaigns across the right combination of channels.
What is MTA?
Multitouch attribution is a marketing metric that assigns credit for sales to multiple touchpoints across the customer journey. It tracks all touchpoints, like paid search ads and email offers, that individuals interact with before making a purchase. Unlike single-touch attribution models, which assign all credit to one touchpoint -- typically the first or last -- MTA helps marketers understand the role of multiple touchpoints.
Common MTA models include the following:
- U-shaped. Assigns the first and last touchpoints the most value.
- Time decay. Assigns more credit to touchpoints that occur closer to the time of sale.
- Data driven. Uses machine learning (ML) algorithms to assign credit based on deep analysis of customer interactions.
In MTA, each touchpoint typically receives credit as a percentage of the total sale amount. For instance, a U-shaped model might assign 40% credit to the first and last touchpoints, whereas those in the middle of the customer journey might only receive 10%.
To find the right MTA model, marketers must first identify their objectives. For instance, an organization that wants to boost brand awareness with its campaign might benefit from a model that gives a lot of credit to the first touchpoint, such as a U-shaped model.
Additionally, organizations can analyze interaction and sales data to determine which touchpoints most significantly contribute to sales. For example, if analysis shows the likelihood of conversion generally increases with continued engagement, the organization might choose a time-decay model. Over time, marketers can test and compare different models to refine their approach.
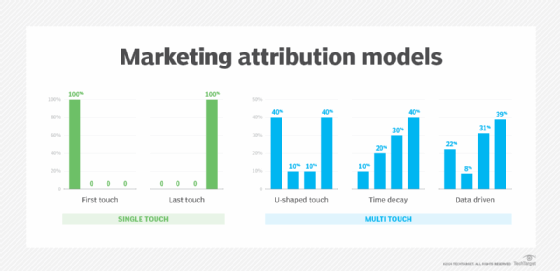
What is MMM?
Marketing mix modeling is a technique marketers use to evaluate past activities and predict the outcomes of future marketing strategies. These models let marketers run simulations to assess how potential marketing tactics will perform in different scenarios.
This technique requires marketers to collect and analyze historical data from the marketing mix, or the four P's, which are the following:
- Product. This refers to the goods and services an organization offers. MMM analyzes how different features, variations and the release of new products affected sales in the past.
- Price. MMM analyzes how past pricing strategies and discounts influenced customer behavior and sales.
- Promotion. This includes all marketing activities, such as email campaigns, TV ads and social media posts. MMM analyzes how these activities affected sales.
- Place. MMM evaluates how the availability of products across different channels and geographic regions affected sales.
To gather this data, marketers rely on internal sources, such as CRM systems and marketing automation platforms. These systems store sales records, marketing spend data and customer engagement metrics, which organizations can use to build MMM models. MMM also factors in external data, like economic conditions and seasonality, which marketers can collect from third-party vendors and public sources, such as the U.S. Census Bureau and World Bank. Data scientists and marketing experts with statistical modeling experience then use advanced analysis techniques, such as regression analysis and ML, to build the models.
4 differences between MTA and MMM
Both techniques can help marketers improve budget allocation, but they differ in areas like focus and data requirements.
1. Focus and perspective
MTA and MMM help marketers measure and improve their strategies, but MTA offers a more granular focus. For example, MTA narrowly focuses on how individual customer interactions, like clicks on social media ads and email opens, contribute to conversions within digital campaigns. It relies on user data to track individual customers across a buyer journey, helping marketers determine which touchpoints influence sales the most.
MMM, on the other hand, offers a broader view of how an organization's overall marketing strategy affects revenue. It analyzes data from diverse sources, such as print ads, digital campaigns, pricing strategies and macroeconomic factors. This mix of online, offline and external data sources offers a more holistic view of how different marketing activities contribute to sales.
2. Data requirements
As MTA offers a granular view of customer behavior, it requires organizations to track user data across devices and platforms, such as clicks, page views, impressions and interactions.
To collect this data, MTA models rely on the following tracking technologies:
- First-party cookies. Small data files that website owners use to track preferences and interactions on their sites and apps.
- Third-party cookies. Data files that track users across different websites and apps.
- Urchin tracking modules. Snippets of text at the end of URLs that track the performance of digital marketing campaigns.
In addition to these tools, marketers can collect data from other sources, such as CRM systems, social media platforms and email marketing systems, to track user interactions. Due to MTA's granularity, it also requires marketers to manage large volumes of data.
MMM, on the other hand, uses aggregated data rather than user-level specifics. It requires marketers to collect data at the channel or campaign level, focusing on broad metrics like ad spend, price changes and customer acquisition cost. It also requires marketers to factor in external data, such as inflation rates, weather patterns and holidays, that might affect sales.
3. Customer privacy
MTA's reliance on tracking tools, like urchin tracking modules and first- and third-party cookies, raises more privacy concerns than MMM.
MTA saw increased adoption around 2010 to help marketers manage the complexities of omnichannel strategies. Yet, demand for stricter privacy led to regulations like the EU's GDPR and California's CCPA, which limit how organizations can collect and process customer data online. These regulations impose strict consent and transparency requirements, which have negatively affected MTA's effectiveness.
MMM offers a more privacy-conscious approach to marketing, because it doesn't track specific data. Instead, it analyzes data from multiple sources, like marketing channels and external factors. Increased consumer demand for data privacy laws, in addition to advancements in AI automation, have led to an MMM resurgence.
4. Time frame
MTA models typically require a few weeks' worth of user data to offer effective insights. This relatively brief time frame lets marketers quickly analyze campaign effectiveness in fast-paced digital marketing settings.
The data collection period for MMM can take anywhere from six months to several years. However, this extended time frame lets marketers include a wider range of variables, such as economic downturns, seasonal changes and shifts in customer preferences, in their models.
How to choose between MTA and MMM
MTA and MMM can improve organizations' marketing efforts, but marketers might find one more helpful than the other, depending on their specific requirements.
Organizations with intricate customer journeys, such as B2B software vendors and financial services companies, can benefit from MTA, because it helps brands better understand how different touchpoints contribute to sales. This approach can also benefit marketers on a budget, because MTA generally costs less than MMM due to its narrower scope and lack of statistical analysis.
MMM, on the other hand, can benefit organizations that have complex marketing strategies spanning online and offline channels, including consumer packaged goods companies and retail chains. Yet, this strategy requires investment in data scientists to build statistical models, which can increase the cost.
Marketers can also use the models together, if they have the budget for it, to form a unified marketing measurement strategy. This strategy offers the granular insights of MTA in addition to the long-term strategic insights of MMM.
Tim Murphy is associate site editor for TechTarget's Customer Experience and Content Management sites.