How do big data and AI work together?
Enterprises are leaning on big data to train AI algorithms and, in turn, are using AI to understand big data. The results are pushing business operations forward.
During the past decade, enterprises built up massive stores of information on everything from business processes to inventory stats. This was the big data revolution.
But simply storing and managing big data isn't enough for organizations to get the most value from all that information. As companies master big data management, forward-thinking ones are applying increasingly intelligent or advanced forms of big data analytics to extract even more value from that information. In particular, they are applying the latest AI and machine learning techniques, which can spot patterns and provide cognitive capabilities across large volumes of data, giving these organizations the ability to apply the next level of analytics needed to extract value from their data.
Furthermore, generative AI systems are increasingly being adopted across organizations to add greater value to data, providing conversational approaches for data analysis and augmentation and adding the ability to gain significant insights from information otherwise trapped in data stores.
How are AI and big data related?
Using machine learning algorithms for big data is a logical step for companies looking to maximize the potential of big data. Machine learning systems use data-driven algorithms and statistical models to analyze and find patterns in data. This is different from traditional rules-based approaches that follow explicit instructions. Big data provides the raw material by which machine learning systems can derive insights. Many organizations are now realizing the benefit of combining big data and machine learning. However, in order for companies to fully utilize the power of both big data and machine learning, it's important to have an understanding of what each can do on its own.
This article is part of
A guide to artificial intelligence in the enterprise
Understanding big data
Big data embodies the idea of extracting and analyzing information from large quantities of data. However, the quantity of data, or its volume, is just one of the considerations in dealing with big data. There are many other important "Vs" of big data that enterprises need to deal with including velocity, variety, veracity, validity, visualization and value.
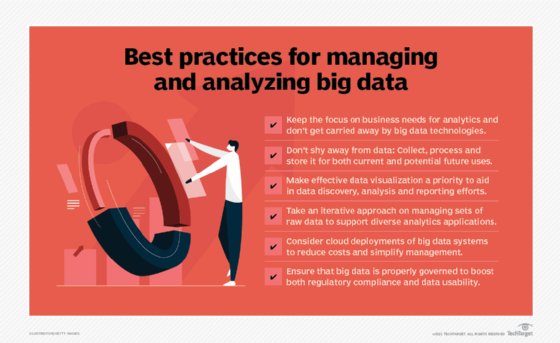
Understanding machine learning
Machine learning, the cornerstone of modern AI applications, provides considerable value to big data applications by deriving higher level insights from big data. Machine learning systems are able to learn and adapt over time without following explicit instructions or programmed code. These machine learning systems use statistical models to analyze and draw inferences from patterns in data. In the past, companies built complex, rules-based systems for a vast range of reporting needs, but found these solutions were brittle and unable to handle continual changes. Now, with the power of machine learning and deep learning companies are able to have systems learn on their big data, improving decision-making, business intelligence and predictive analysis over time.
Machine learning approaches get their power by virtue of the ability to discover patterns in data. The more data that these machine learning algorithms have access to, the more they can identify patterns in the data and then apply those learned patterns to future data. These patterns can range from recommendation systems to anomaly detection, image and object recognition, to conversational and natural language processing (NLP).
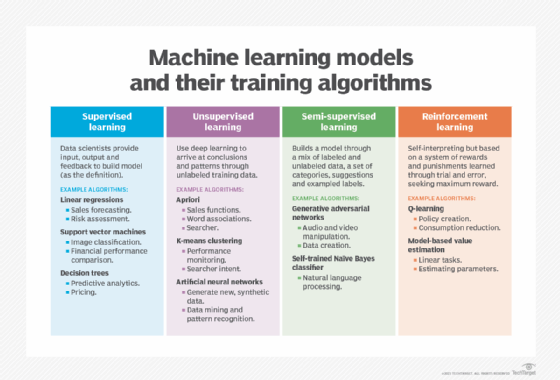
Categories of machine learning algorithms
In general, there are a few categories of machine learning algorithms in broad use:
- Supervised learning approaches learn from patterns identified by human-tagged training data.
- Unsupervised learning approaches learn through the discovery of patterns in the data.
- Reinforcement learning approaches learn through trial-and-error approaches.
The most common supervised learning algorithms include deep learning neural networks, which are the basis of the most powerful machine learning models built today, as well as proven approaches, such as decision tree and random forest algorithms, support vector machines, k-nearest neighbor and Bayesian approaches.
Unsupervised machine learning algorithms, such as k-means clustering, principal component analysis and Gaussian mixture models, are widely used to spot patterns and anomalies in data.
Reinforcement learning approaches, such as Q-learning, state-action-reward-state-action and Deep Q-Learning, are also widely adopted.
The most powerful large language models (LLMs), which form the basis of today's widely used conversational generative AI systems, use many of these methods above, learning patterns from petabytes of training data.
Understanding generative AI
Generative AI applications have proven to be some of the most powerful and widely used applications of AI. Generative AI applies machine learning techniques to the creation of new data based on patterns learned from a large amount of data. Generative AI models are built to interact with users through conversational modes, as they have been trained on a large corpus of internet data that contains many types of human communications, including conversations, interviews, social media posts and more. With these pretrained LLMs, users can access the patterns learned from all that data to generate new text, images, audio or other forms of outputs using natural language prompts to generate these outputs without having to do any coding or building specialized models.
How does AI benefit big data?
AI, coupled with big data, is impacting businesses across a variety of sectors and industries. Some of the benefits include the following:
- 360-degree view of the customer. Our digital footprints are growing at an astounding rate and companies are using this to their advantage to provide greater insights into each individual. Companies used to move data into and out of data warehouses and create static reports that take a long time to generate and even longer to modify. Now, smart organizations are utilizing distributed, automated and intelligent analytics tools that sit on top of data lakes designed to collect and synthesize data from disparate sources at once. This is transforming the way companies understand their customers.
- Conversational interaction with data. Generative AI applications, as noted, are enabling users to have interactive conversations with data and systems. In the past, extracting value from data required sophisticated database skills and knowledge, but with the increasing widespread use of generative AI systems, even the most casual user can get significant value from their data by engaging in back-and-forth conversational prompts.
- Hyperpersonalization and recommendation systems. AI systems can develop a profile of individual user behavior and then apply that profile to learn and adapt over time for a wide variety of purposes, including displaying relevant content, recommending relevant products, providing personalized recommendations and similar uses. In this way, AI-powered systems can create personalized recommendations based on user interaction patterns and searches. Industries as varied as marketing, education, finance and healthcare are making good use of these AI-powered profiles to deliver personalized products, learning, healthcare and financial solutions.
- Improved forecasting and price optimization. Traditionally, companies base their estimate of current year's sales on data from the prior year. However, due to a variety of factors such as changing trends, global pandemics or other hard-to-predict factors, forecasting and price optimization can be quite difficult with traditional approaches. Big data is giving organizations the power to spot patterns and trends early and know how those trends will impact future performance. It's helping companies make better decisions by giving organizations more information about what could potentially happen in the future with greater likelihood. Companies using big data and AI-based approaches, especially in retail, are able to improve seasonal forecasting, reducing errors by as much as 50 percent.
- Improved customer acquisition and retention. With big data and AI, organizations have a better handle on what their customers are interested in, how products and services are being used and reasons why customers stop purchasing or using their offerings. Through big data applications, companies can more accurately identify what customers are really looking for and observe their behavioral patterns. They can then apply those patterns to improve products, generate better conversions, improve brand loyalty, spot trends earlier or find additional ways to improve overall customer satisfaction.
- Cybersecurity and fraud prevention. Tackling fraud is a never-ending battle for businesses of all shapes and sizes. Organizations using big data-powered analytics to identify patterns of fraud are able to detect anomalies in system behavior and thwart bad actors. Big data systems have the power to comb through very large quantities of data from transactional or log data, databases and files to identify, prevent, detect and mitigate potential fraudulent behavior. These systems can also combine a variety of data types including both internal and external data to alert companies to cybersecurity threats that haven't yet shown up in their own systems. Without big data processing and analysis capabilities, this would be impossible.
- Identifying and mitigating potential risks. Anticipating, planning and responding to constant changes and risks is critical to the longevity of any business. Big data is proving its value in the risk management arena, providing early visibility to potential risks, helping to quantify the exposure to risks and potential losses, and expedite changes. Big data-powered models are also helping organizations identify and address customer and market risks as well as challenges emerging from unpredicted events such as natural disasters. Companies can digest information from disparate data sources and synthesize the information to provide greater situational awareness and understanding of how to allocate people or resources to deal with emerging threats.
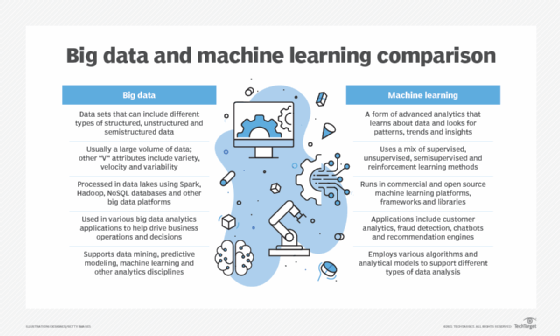
How does AI improve insight into data?
Big data and machine learning aren't really competing concepts and, when combined, they provide the opportunity for some incredible results. Emerging big data approaches are giving organizations powerful ways to store, manage, process and make sense of their data. Machine learning systems learn from that data. In fact, successfully dealing with the various "Vs" of big data will help make machine learning models more accurate and powerful. Machine learning models learn from data and translate these insights to help improve business operations. Likewise, big data management approaches improve machine learning systems by giving these models the large quantity of high quality, relevant data needed to build those models.
The amount of data generated will continue to grow at an astounding rate. By 2030, UBS research predicted that worldwide data will grow to over 660 zettabytes of data -- equivalent to 610 iPhones worth of data per person in the world. As enterprises continue to store huge volumes of data, the only way they will even possibly be able to make sense of it is with the help of machine learning. The machine learning process will come to rely heavily on big data and companies that do not leverage machine learning will be left behind.
Examples of AI and big data
Many organizations have discovered the power of machine learning-enhanced big data analytics and are using the power of big data and AI in a variety of ways:
- Netflix uses machine learning algorithms to help better understand each individual user, providing more personalized recommendations. This keeps the user on their platform for longer and creates a more positive overall customer experience.
- Mayo Clinic applies AI approaches to big data to improve patient care. Through the use of machine learning, Mayo Clinic analyzes electronic health records to identify patterns and trends that can help predict risks to patients, susceptibility to disease and other aspects that can improve patient care.
- Google uses machine learning to provide users with a highly valuable and personalized experience. The company uses machine learning in a variety of products, including providing predictive text in emails and optimized directions for users looking to get to a designated location. Google is also using its massive amounts of big data in the development of generative AI LLMs that are used to enhance the search experience.
- Starbucks is using the power of big data, AI and NLP to provide personalized emails based on data from customers' past purchases. Rather than crafting only a few dozen emails on a monthly basis with offers for the broad Starbucks audience, Starbucks is using its "digital flywheel" with AI-enabled capabilities to generate over 400,000 personalized weekly emails featuring different promotions and offers.
- Amazon has long been known to use AI and big data to create hyperpersonalized shopping experiences for customers. Through the analysis of customer behavior, purchase history and browsing patterns, Amazon's recommendation engine suggests products that users might be interested in purchasing, enhancing the shopping experience and boosting sales.
- Spotify uses AI and big data to provide tailored music recommendations to its users. The platform analyzes listening habits for each individual user. This includes the songs they play, songs they skip and saved songs, which then are used to curate custom playlists. This data-driven personalization keeps users engaged and on the platform.
- The Transportation Security Administration, in collaboration with U.S. Customs and Border Protection, is using big data-powered AI facial recognition technology to help with the identity and boarding pass verification process at checkpoints.These AI-enhanced systems are speeding up the verification process, while maintaining the required high degrees of accuracy.
Companies are going to continue to combine the power of machine learning, big data, visualization tools and analytics to help their businesses with decision-making through the analysis of raw data.
Without big data, none of these more personalized experiences would be possible. In the years ahead it will be no surprise that companies that do not combine big data and AI will have a hard time meeting their digital transformation needs and be left behind.
Editor's note: This article was published in 2023 and expanded to include new use cases for AI and big data and infomration about generative AI.
Ron Schmelzer is managing partner and founder of Cognilytica.
Kathleen Walch is managing partner and founder of Cognilytica.